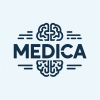
Modelling and vErification of alkaptonuria and multiple sclerosis Driven by biomedICAl data
The goal of this project is to bridge the gap between data, models and validation, integrating the data-driven and model-driven research directions, so that to develop next generation modelling and analysis approaches for biomedical investigations.
We are developing the MEDICA framework, which combines three analysis phases: (1) extracting existing knowledge from integrated datasets, that will feed into (2) building multilevel mechanistic models, which will then go through (3) validation and model checking.
Biomedical research needs are the driving force of the project. We are tackling open research questions for Multiple Sclerosis (MS) and Alkaptonuria (AKU), for which we have world-leading experts in our consortium.
Our team
- Vincenzo Lomonaco
- Silvia Giulia Galfrè
- Francesca Levi
- Francesco Massafra
- Paolo Milazzo
- Giang Pham
- Corrado Priami
- Samuele Punzo
- Alina Sîrbu (UNIBO)
- Elvio Amparore
- Marco Beccuti
- Marinella Clerico
- Massimiliano De Pierro
- Alessandro Maglione
- Simone Pernice
- Simona Rolla
- James Sproston
- Moreno Falaschi
- Francesca Ariani
- Linda Brodo
- Sara Brunetti
- Caterina Graziani
- Veronica Lachi
- Giulia Palma
- Alessandra Renieri
- Simone Rinaldi
- Bianca Roncaglia
- Ottavia Spiga
- Anna Visibelli
- Simone Tini
- Ruggero Lanotte
- Desiree Manicardi
- Nicoletta Sabadini
Contact: alina.sirbu@unipi.it
Publications
2025
Pham, Giang; Milazzo, Paolo
Gene Importance Assessment based on Shapley Values for Boolean Networks: Validation and Scalability Analysis Proceedings Article
In: International Symposium ``from Data to Models and Back'' (DataMod 2024), Springer 2025.
@inproceedings{pham2025preliminary,
title = {Gene Importance Assessment based on Shapley Values for Boolean Networks: Validation and Scalability Analysis},
author = {Giang Pham and Paolo Milazzo},
year = {2025},
date = {2025-01-01},
urldate = {2025-01-01},
booktitle = {International Symposium ``from Data to Models and Back'' (DataMod 2024)},
volume = {LNCS 15556},
organization = {Springer},
keywords = {},
pubstate = {published},
tppubtype = {inproceedings}
}
2024
Graziani, Caterina; Drucks, Tamara; Jogl, Fabian; Bianchini, Monica; scarselli,; Gärtner, Thomas
The Expressive Power of Path-Based Graph Neural Networks Proceedings Article
In: Forty-first International Conference on Machine Learning, 2024.
@inproceedings{graziani2024the,
title = {The Expressive Power of Path-Based Graph Neural Networks},
author = {Caterina Graziani and Tamara Drucks and Fabian Jogl and Monica Bianchini and scarselli and Thomas Gärtner},
url = {https://openreview.net/forum?id=io1XSRtcO8},
year = {2024},
date = {2024-01-01},
urldate = {2024-01-01},
booktitle = {Forty-first International Conference on Machine Learning},
abstract = {We systematically investigate the expressive power of path-based graph neural networks. While it has been shown that path-based graph neural networks can achieve strong empirical results, an investigation into their expressive power is lacking. Therefore, we propose PATH-WL, a general class of color refinement algorithms based on paths and shortest path distance information. We show that PATH-WL is incomparable to a wide range of expressive graph neural networks, can count cycles, and achieves strong empirical results on the notoriously difficult family of strongly regular graphs. Our theoretical results indicate that PATH-WL forms a new hierarchy of highly expressive graph neural networks.},
keywords = {},
pubstate = {published},
tppubtype = {inproceedings}
}
Brodo, Linda; Bruni, Roberto; Falaschi, Moreno
A framework for monitored dynamic slicing of Reaction Systems Journal Article
In: Natural Computing, 2024.
@article{BBF24,
title = {A framework for monitored dynamic slicing of Reaction Systems},
author = {Linda Brodo and Roberto Bruni and Moreno Falaschi},
doi = {https://doi.org/10.1007/s11047-024-09976-3},
year = {2024},
date = {2024-01-01},
urldate = {2024-01-01},
journal = {Natural Computing},
abstract = {Reaction systems (RSs) are a computational framework inspired by biochemical mechanisms. A RS defines a finite set of reactions over a finite set of entities. Typically each reaction has a local scope, because it is concerned with a small set of entities, but complex models can involve a large number of reactions and entities, and their computation can manifest unforeseen emerging behaviours. When a deviation is detected, like the unexpected production of some entities, it is often difficult to establish its causes, e.g., which entities were directly responsible or if some reaction was misconceived. Slicing is a well-known technique for debugging, which can point out the program lines containing the faulty code. In this paper, we define the first dynamic slicer for RSs and show that it can help to detect the causes of erroneous behaviour and highlight the involved reactions for a closer inspection. To fully automate the debugging process, we propose to distil monitors for starting the slicing whenever a violation from a safety specification is detected. We have integrated our slicer in BioResolve, written in Prolog which provides many useful features for the formal analysis of RSs. We define the slicing algorithm for basic RSs and then enhance it for dealing with quantitative extensions of RSs, where timed processes and linear processes can be represented. Our framework is shown at work on suitable biologically inspired RS models.},
keywords = {},
pubstate = {published},
tppubtype = {article}
}
Castiglioni, Valentina; Loreti, Michele; Tini, Simone
Bio-Stark: A Tool for the Time-Point Robustness Analysis of Biological Systems Proceedings Article
In: Gori, Roberta; Milazzo, Paolo; Tribastone, Mirco (Ed.): Computational Methods in Systems Biology - 22nd International Conference, CMSB 2024, Pisa, Italy, September 16-18, 2024, Proceedings, pp. 62–70, Springer, 2024.
@inproceedings{DBLP:conf/cmsb/CastiglioniLT24,
title = {Bio-Stark: A Tool for the Time-Point Robustness Analysis of Biological Systems},
author = {Valentina Castiglioni and Michele Loreti and Simone Tini},
editor = {Roberta Gori and Paolo Milazzo and Mirco Tribastone},
url = {https://doi.org/10.1007/978-3-031-71671-3_5},
doi = {10.1007/978-3-031-71671-3_5},
year = {2024},
date = {2024-01-01},
urldate = {2024-01-01},
booktitle = {Computational Methods in Systems Biology - 22nd International Conference,
CMSB 2024, Pisa, Italy, September 16-18, 2024, Proceedings},
volume = {14971},
pages = {62–70},
publisher = {Springer},
series = {Lecture Notes in Computer Science},
keywords = {},
pubstate = {published},
tppubtype = {inproceedings}
}
Castiglioni, Valentina; Loreti, Michele; Tini, Simone
RobTL: Robustness Temporal Logic for CPS Proceedings Article
In: Majumdar, Rupak; Silva, Alexandra (Ed.): 35th International Conference on Concurrency Theory, CONCUR 2024, September 9-13, 2024, Calgary, Canada, pp. 15:1–15:23, Schloss Dagstuhl - Leibniz-Zentrum für Informatik, 2024.
@inproceedings{DBLP:conf/concur/CastiglioniLT24,
title = {RobTL: Robustness Temporal Logic for CPS},
author = {Valentina Castiglioni and Michele Loreti and Simone Tini},
editor = {Rupak Majumdar and Alexandra Silva},
url = {https://doi.org/10.4230/LIPIcs.CONCUR.2024.15},
doi = {10.4230/LIPICS.CONCUR.2024.15},
year = {2024},
date = {2024-01-01},
urldate = {2024-01-01},
booktitle = {35th International Conference on Concurrency Theory, CONCUR 2024,
September 9-13, 2024, Calgary, Canada},
volume = {311},
pages = {15:1–15:23},
publisher = {Schloss Dagstuhl - Leibniz-Zentrum für Informatik},
series = {LIPIcs},
keywords = {},
pubstate = {published},
tppubtype = {inproceedings}
}
Castiglioni, Valentina; Lanotte, Ruggero; Loreti, Michele; Tini, Simone
Evaluating the Effectiveness of Digital Twins Through Statistical Model Checking with Feedback and Perturbations Proceedings Article
In: Haxthausen, Anne E.; Serwe, Wendelin (Ed.): Formal Methods for Industrial Critical Systems - 29th International Conference, FMICS 2024, Milan, Italy, September 9-11, 2024, Proceedings, pp. 21–39, Springer, 2024.
@inproceedings{DBLP:conf/fmics/CastiglioniLLT24,
title = {Evaluating the Effectiveness of Digital Twins Through Statistical Model Checking with Feedback and Perturbations},
author = {Valentina Castiglioni and Ruggero Lanotte and Michele Loreti and Simone Tini},
editor = {Anne E. Haxthausen and Wendelin Serwe},
url = {https://doi.org/10.1007/978-3-031-68150-9_2},
doi = {10.1007/978-3-031-68150-9_2},
year = {2024},
date = {2024-01-01},
urldate = {2024-01-01},
booktitle = {Formal Methods for Industrial Critical Systems - 29th International
Conference, FMICS 2024, Milan, Italy, September 9-11, 2024, Proceedings},
volume = {14952},
pages = {21–39},
publisher = {Springer},
series = {Lecture Notes in Computer Science},
keywords = {},
pubstate = {published},
tppubtype = {inproceedings}
}
Castiglioni, Valentina; Lanotte, Ruggero; Loreti, Michele; Manicardi, Desiree; Tini, Simone
Robustness for biochemical networks: Step-by-step approach Journal Article
In: Theoretical Computer Science, vol. 1022, pp. 114934, 2024, ISSN: 0304-3975.
@article{CASTIGLIONI2024114934,
title = {Robustness for biochemical networks: Step-by-step approach},
author = {Valentina Castiglioni and Ruggero Lanotte and Michele Loreti and Desiree Manicardi and Simone Tini},
url = {https://www.sciencedirect.com/science/article/pii/S0304397524005516},
doi = {https://doi.org/10.1016/j.tcs.2024.114934},
issn = {0304-3975},
year = {2024},
date = {2024-01-01},
urldate = {2024-01-01},
journal = {Theoretical Computer Science},
volume = {1022},
pages = {114934},
keywords = {},
pubstate = {published},
tppubtype = {article}
}
Brodo, Linda; Bruni, Roberto; Falaschi, Moreno; Gori, Roberta; Milazzo, Paolo; Montagna, Valeria; Pulieri, Pasquale
Causal analysis of positive Reaction Systems Journal Article
In: International Journal on Software Tools for Technology Transfer, pp. 1–18, 2024.
@article{brodo2024causal,
title = {Causal analysis of positive Reaction Systems},
author = {Linda Brodo and Roberto Bruni and Moreno Falaschi and Roberta Gori and Paolo Milazzo and Valeria Montagna and Pasquale Pulieri},
year = {2024},
date = {2024-01-01},
urldate = {2024-01-01},
journal = {International Journal on Software Tools for Technology Transfer},
pages = {1–18},
publisher = {Springer},
keywords = {},
pubstate = {published},
tppubtype = {article}
}
Bruni, Roberto; Gori, Roberta; Milazzo, Paolo; Siboulet, Hélène
Melding Boolean networks and reaction systems under synchronous, asynchronous and most permissive semantics Journal Article
In: Natural Computing, vol. 23, no. 2, pp. 235–267, 2024.
@article{bruni2024melding,
title = {Melding Boolean networks and reaction systems under synchronous, asynchronous and most permissive semantics},
author = {Roberto Bruni and Roberta Gori and Paolo Milazzo and Hélène Siboulet},
year = {2024},
date = {2024-01-01},
urldate = {2024-01-01},
journal = {Natural Computing},
volume = {23},
number = {2},
pages = {235–267},
publisher = {Springer},
keywords = {},
pubstate = {published},
tppubtype = {article}
}
Bowles, Juliana; Brodo, Linda; Bruni, Roberto; Falaschi, Moreno; Gori, Roberta; Milazzo, Paolo
Enhancing Reaction Systems with Guards for Analysing Comorbidity Treatment Strategies Proceedings Article
In: International Conference on Computational Methods in Systems Biology (CMSB 2024), pp. 27–44, Springer 2024.
@inproceedings{bowles2024enhancing,
title = {Enhancing Reaction Systems with Guards for Analysing Comorbidity Treatment Strategies},
author = {Juliana Bowles and Linda Brodo and Roberto Bruni and Moreno Falaschi and Roberta Gori and Paolo Milazzo},
year = {2024},
date = {2024-01-01},
urldate = {2024-01-01},
booktitle = {International Conference on Computational Methods in Systems Biology (CMSB 2024)},
volume = {LNCS 14971},
pages = {27–44},
organization = {Springer},
keywords = {},
pubstate = {published},
tppubtype = {inproceedings}
}
Ballis, Demis; Brodo, Linda; Falaschi, Moreno; Olarte, Carlos
ccReact: a rewriting framework for the formal analysis of reaction systems Journal Article
In: International Journal on Software Tools for Technology Transfer, vol. 26, pp. 707–725, 2024.
@article{BBFO24,
title = {ccReact: a rewriting framework for the formal analysis of reaction systems},
author = {Demis Ballis and Linda Brodo and Moreno Falaschi and Carlos Olarte},
url = {https://doi.org/10.1007/s10009-024-00772-z},
doi = {10.1007/s10009-024-00772-z},
year = {2024},
date = {2024-01-01},
urldate = {2024-01-01},
journal = {International Journal on Software Tools for Technology Transfer},
volume = {26},
pages = {707–725},
publisher = {Springer},
keywords = {},
pubstate = {published},
tppubtype = {article}
}
Ballis, Demis; Brodo, Linda; Falaschi, Moreno
Modeling and Analyzing Reaction Systems in Maude Journal Article
In: Electronics, vol. 13, no. 6, 2024.
@article{BBF24b,
title = {Modeling and Analyzing Reaction Systems in Maude},
author = {Demis Ballis and Linda Brodo and Moreno Falaschi},
url = {https://doi.org/10.3390/electronics13061139},
doi = {10.3390/electronics13061139},
year = {2024},
date = {2024-01-01},
urldate = {2024-01-01},
journal = {Electronics},
volume = {13},
number = {6},
publisher = {MDPI},
keywords = {},
pubstate = {published},
tppubtype = {article}
}
Bendjeddou, Asma; Brodo, Linda; Falaschi, Moreno; Tiezzi, Elisa Benedetta Primavera
A Computational Model of the Secondary Hemostasis Pathway in Reaction Systems Journal Article
In: Mathematics, vol. 12, no. 15, 2024.
@article{DBLP:journals/nc/BrodoBF24,
title = {A Computational Model of the Secondary Hemostasis Pathway in Reaction Systems},
author = {Asma Bendjeddou and Linda Brodo and Moreno Falaschi and Elisa Benedetta Primavera Tiezzi},
url = {https://doi.org/10.3390/math12152422},
doi = {10.3390/math12152422},
year = {2024},
date = {2024-01-01},
urldate = {2024-01-01},
journal = {Mathematics},
volume = {12},
number = {15},
publisher = {MDPI},
keywords = {},
pubstate = {published},
tppubtype = {article}
}
Ballis, Demis; Brodo, Linda; Falaschi, Moreno; Olarte, Carlos
Process Calculi and Rewriting Techniques for Analyzing Reaction Systems Proceedings Article
In: Gori, Roberta; Milazzo, Paolo; Tribastone, Mirco (Ed.): Computational Methods in Systems Biology - 22nd International Conference, CMSB 2024, Pisa, Italy, September 16-18, 2024, Proceedings, pp. 1–18, Springer, 2024.
@inproceedings{DBLP:conf/cmsb/BallisBFO24,
title = {Process Calculi and Rewriting Techniques for Analyzing Reaction Systems},
author = {Demis Ballis and Linda Brodo and Moreno Falaschi and Carlos Olarte},
editor = {Roberta Gori and Paolo Milazzo and Mirco Tribastone},
url = {https://doi.org/10.1007/978-3-031-71671-3_1},
doi = {10.1007/978-3-031-71671-3_1},
year = {2024},
date = {2024-01-01},
urldate = {2024-01-01},
booktitle = {Computational Methods in Systems Biology - 22nd International Conference,
CMSB 2024, Pisa, Italy, September 16-18, 2024, Proceedings},
volume = {14971},
pages = {1–18},
publisher = {Springer},
series = {Lecture Notes in Computer Science},
keywords = {},
pubstate = {published},
tppubtype = {inproceedings}
}
Brodo, Linda; Bruni, Roberto; Falaschi, Moreno
A framework for monitored dynamic slicing of reaction systems Journal Article
In: Nat. Comput., vol. 23, no. 2, pp. 217–234, 2024.
@article{DBLP:journals/nc/BrodoBF24b,
title = {A framework for monitored dynamic slicing of reaction systems},
author = {Linda Brodo and Roberto Bruni and Moreno Falaschi},
url = {https://doi.org/10.1007/s11047-024-09976-3},
doi = {10.1007/S11047-024-09976-3},
year = {2024},
date = {2024-01-01},
urldate = {2024-01-01},
journal = {Nat. Comput.},
volume = {23},
number = {2},
pages = {217–234},
keywords = {},
pubstate = {published},
tppubtype = {article}
}
Sproston, Jeremy
Clock-Dependent Probabilistic Timed Automata with One Clock and No Memory Proceedings Article
In: Ogata, Kazuhiro; Méry, Dominique; Sun, Meng; Liu, Shaoying (Ed.): Proceedings of the 25th International Conference on Formal Engineering Methods (ICFEM 2024), pp. 70–84, Springer, 2024.
@inproceedings{DBLP:conf/icfem/Sproston24,
title = {Clock-Dependent Probabilistic Timed Automata with One Clock and No Memory},
author = {Jeremy Sproston},
editor = {Kazuhiro Ogata and Dominique Méry and Meng Sun and Shaoying Liu},
doi = {10.1007/978-981-96-0617-7_5},
year = {2024},
date = {2024-01-01},
urldate = {2024-01-01},
booktitle = {Proceedings of the 25th International Conference
on Formal Engineering Methods (ICFEM 2024)},
volume = {15394},
pages = {70–84},
publisher = {Springer},
series = {Lecture Notes in Computer Science},
keywords = {},
pubstate = {published},
tppubtype = {inproceedings}
}
Graziani, Caterina; Drucks, Tamara; Jogl, Fabian; Bianchini, Monica; scarselli,; Gärtner, Thomas
The Expressive Power of Path-Based Graph Neural Networks Proceedings Article
In: Forty-first International Conference on Machine Learning, 2024.
@inproceedings{graziani2024the,
title = {The Expressive Power of Path-Based Graph Neural Networks},
author = {Caterina Graziani and Tamara Drucks and Fabian Jogl and Monica Bianchini and scarselli and Thomas Gärtner},
url = {https://openreview.net/forum?id=io1XSRtcO8},
year = {2024},
date = {2024-01-01},
urldate = {2024-01-01},
booktitle = {Forty-first International Conference on Machine Learning},
keywords = {},
pubstate = {published},
tppubtype = {inproceedings}
}
2023
Brodo, Linda; Bruni, Roberto; Falaschi, Moreno; Gori, Roberta; Milazzo, Paolo
Attractor and Slicing Analysis of a T Cell Differentiation Model Based on Reaction Systems Proceedings Article
In: International Symposium ``from Data to Models and Back'' (DataMod 2023), Springer 2023.
@inproceedings{brodo2024attractor,
title = {Attractor and Slicing Analysis of a T Cell Differentiation Model Based on Reaction Systems},
author = {Linda Brodo and Roberto Bruni and Moreno Falaschi and Roberta Gori and Paolo Milazzo},
year = {2023},
date = {2023-11-07},
booktitle = {International Symposium ``from Data to Models and Back'' (DataMod 2023)},
volume = {LNCS},
organization = {Springer},
keywords = {},
pubstate = {published},
tppubtype = {inproceedings}
}
Pham, Giang; Milazzo, Paolo
Preliminary Results on Shapley Value Notions and Propagation Methods for Boolean Networks Proceedings Article
In: International Symposium ``from Data to Models and Back'' (DataMod 2023), Springer 2023.
@inproceedings{pham2025preliminaryb,
title = {Preliminary Results on Shapley Value Notions and Propagation Methods for Boolean Networks},
author = {Giang Pham and Paolo Milazzo},
year = {2023},
date = {2023-11-07},
booktitle = {International Symposium ``from Data to Models and Back'' (DataMod 2023)},
volume = {LNCS},
organization = {Springer},
keywords = {},
pubstate = {published},
tppubtype = {inproceedings}
}
Pernice, Simone; Maglione, Alessandro; Tortarolo, Dora; Sirovich, Roberta; Clerico, Marinella; Rolla, Simona; Beccuti, Marco; Cordero, Francesca
A new computational workflow to guide personalized drug therapy Journal Article
In: Journal of Biomedical Informatics, vol. 148, pp. 104546, 2023, ISSN: 1532-0464.
@article{PERNICE2023104546,
title = {A new computational workflow to guide personalized drug therapy},
author = {Simone Pernice and Alessandro Maglione and Dora Tortarolo and Roberta Sirovich and Marinella Clerico and Simona Rolla and Marco Beccuti and Francesca Cordero},
url = {https://www.sciencedirect.com/science/article/pii/S1532046423002678},
doi = {https://doi.org/10.1016/j.jbi.2023.104546},
issn = {1532-0464},
year = {2023},
date = {2023-01-01},
urldate = {2023-01-01},
journal = {Journal of Biomedical Informatics},
volume = {148},
pages = {104546},
abstract = {Objective:
Computational models are at the forefront of the pursuit of personalized medicine thanks to their descriptive and predictive abilities. In the presence of complex and heterogeneous data, patient stratification is a prerequisite for effective precision medicine, since disease development is often driven by individual variability and unpredictable environmental events. Herein, we present GreatNectorworkflow as a valuable tool for (i) the analysis and clustering of patient-derived longitudinal data, and (ii) the simulation of the resulting model of patient-specific disease dynamics.
Methods:
GreatNectoris designed by combining an analytic strategy composed of CONNECTOR, a data-driven framework for the inspection of longitudinal data, and an unsupervised methodology to stratify the subjects with GreatMod, a quantitative modeling framework based on the Petri Net formalism and its generalizations.
Results:
To illustrate GreatNectorcapabilities, we exploited longitudinal data of four immune cell populations collected from Multiple Sclerosis patients. Our main results report that the T-cell dynamics after alemtuzumab treatment separate non-responders versus responders patients, and the patients in the non-responders group are characterized by an increase of the Th17 concentration around 36 months.
Conclusion:
GreatNectoranalysis was able to stratify individual patients into three model meta-patients whose dynamics suggested insight into patient-tailored interventions.},
keywords = {},
pubstate = {published},
tppubtype = {article}
}
Computational models are at the forefront of the pursuit of personalized medicine thanks to their descriptive and predictive abilities. In the presence of complex and heterogeneous data, patient stratification is a prerequisite for effective precision medicine, since disease development is often driven by individual variability and unpredictable environmental events. Herein, we present GreatNectorworkflow as a valuable tool for (i) the analysis and clustering of patient-derived longitudinal data, and (ii) the simulation of the resulting model of patient-specific disease dynamics.
Methods:
GreatNectoris designed by combining an analytic strategy composed of CONNECTOR, a data-driven framework for the inspection of longitudinal data, and an unsupervised methodology to stratify the subjects with GreatMod, a quantitative modeling framework based on the Petri Net formalism and its generalizations.
Results:
To illustrate GreatNectorcapabilities, we exploited longitudinal data of four immune cell populations collected from Multiple Sclerosis patients. Our main results report that the T-cell dynamics after alemtuzumab treatment separate non-responders versus responders patients, and the patients in the non-responders group are characterized by an increase of the Th17 concentration around 36 months.
Conclusion:
GreatNectoranalysis was able to stratify individual patients into three model meta-patients whose dynamics suggested insight into patient-tailored interventions.
Graziani, Caterina; Drucks, Tamara; Bianchini, Monica; scarselli,; Gärtner, Thomas
No PAIN no Gain: More Expressive GNNs with Paths Proceedings Article
In: NeurIPS 2023 Workshop: New Frontiers in Graph Learning, 2023.
@inproceedings{<LineBreak>graziani2023no,
title = {No PAIN no Gain: More Expressive GNNs with Paths},
author = {Caterina Graziani and Tamara Drucks and Monica Bianchini and scarselli and Thomas Gärtner},
url = {https://openreview.net/forum?id=q2xXh4M9Dx},
year = {2023},
date = {2023-01-01},
urldate = {2023-01-01},
booktitle = {NeurIPS 2023 Workshop: New Frontiers in Graph Learning},
abstract = {Motivated by the lack of theoretical investigation into the discriminative power of paths, we characterize classes of graphs where paths are sufficient to identify every instance. Our analysis motivates the integration of paths into the learning procedure of graph neural networks in order to enhance their expressiveness. We formally justify the use of paths based on finite-variable counting logic and prove the effectiveness of paths to recognize graph structural features related to cycles and connectivity. We show that paths are able to identify graphs for which higher-order models fail. Building on this, we propose PAth Isomorphism Network (PAIN), a novel graph neural network that replaces the topological neighborhood with paths in the aggregation step of the message-passing procedure. This modification leads to an algorithm that is strictly more expressive than the Weisfeiler-Leman graph isomorphism test, at the cost of a polynomial-time step for every iteration and fixed path length. We support our theoretical findings by empirically evaluating PAIN on synthetic datasets.},
keywords = {},
pubstate = {published},
tppubtype = {inproceedings}
}
This project was financed by the European Union – Next-GenerationEU – National Recovery and Resilience Plan (NRRP) – MISSION 4 COMPONENT 2, INVESTMENT N. 1.1, CALL PRIN 2022 D.D. 104 02-02-2022 – MEDICA CUP N. I53D23003720006 .
